The great compromise: Balancing Pricing Transparency and Accuracy

.png)
It seems that actuaries regularly find themselves sitting on a powder keg – in need of satisfying two often polarizing goals that are crucial to the insurance company’s success. On one hand, an actuary wants to predict probabilities of risks occurring and their costs as accurately as possible. However, this is not always feasible due to the very peculiar nature of the insurance business.
Specifically, the fact that translating those accurate predictions and calculations behind it into a transparent price should always be available upon customer request or for regulatory purposes. This restricts the scope of available methods that Pricing teams can utilize for obtaining those premiums.
This article is devoted to describing both challenges in greater detail and introducing state-of-the-art techniques for satisfying this great compromise.
As clear as a crystal …
Insurance business heavily relies on customer relationship & their loyalty which is hard to build and maintain. Pricing transparency helps to ensure that this relationship is honest for all parties involved:
- customers,
- company,
- & the regulators.
A pricing transparency definition provided by ChatGPT is the following:
“Insurance pricing transparency refers to the extent to which insurance companies make information about their pricing practices and factors affecting premiums available to consumers. It is an important issue because insurance pricing can be complex and opaque, making it difficult for consumers to understand how premiums are calculated and whether they are getting a fair price for their coverage.”
And I think it got it right this time!
There is no strict definition on the degree of transparency that should be publicly available, meaning that the standards would vary across different types of insurance companies, jurisdictions, etc. However, some rules would be fairly standard across many countries, such as the factors that cannot be used in determining insurance premiums. One example would be the use of gender as a pricing factor. In Europe it was banned by the European Union's Gender Directive [1], which came into effect on December 21, 2012 and applied to all European Union member states. The Affordable Care Act (ACA) [2] that came live in 2014 had a very similar effect on the American health insurance market.
Besides those there are actuarial standards that some actuaries must comply with. In the UK, one of the main regulatory frameworks is the Treating Customers Fairly (TCF) [3] introduced by the UK Financial Conduct Authority (FCA) in 2007. One of its key principles is the concept of Policyholder’s Reasonable Expectations (PRE). PRE aims to ensure that policyholders are not misled or unfairly treated by the terms and conditions of their insurance policies, and that they understand the coverage they are purchasing.
The last statement means that any policy terms that may be considered unusual or surprising must be clearly stated. On top of that it is a convenient introduction to the topic of Fairness which might be subject to a separate blog post soon. Lack of compliance with TCF (and PRE) & other principles might result in fines, legal actions, and other penalties as well as substantial reputational damage and loss of customer trust.
The Pricing Transparency is becoming an increasingly important topic from both regulatory and academic points of view. The recently published Supervisory statement on differential pricing practices in non-life insurance lines of business [4] from EIOPA further proves that regulators are keeping an eye on pricing strategies that might potentially breach TCF or other similar principles. Some well-known actuarial experts are also trying to tackle this problem in their research. For curious readers, references to those papers will be provided below [5].
The reader might be wondering:
“If Pricing Transparency is such an important topic, how can we even think about using complex statistical models to calculate premiums?”
Please, fasten your seat belts, because we are getting there!
… or right on the button?
Maintaining a sufficient degree of Pricing Transparency does not mean that its Accuracy is of lesser importance. Both items are equally important and have to be considered simultaneously. The 21st century thus far has been a period of rapid development in the field of statistics which resulted in significant improvements in predictive power of the available estimators. The biggest caveat is that with great power comes great responsibility (however, it does not mean that Spider-Man predicted actuarial struggles with this quote!) and explaining those predictions easily has also become a bigger challenge.
With everything in mind, the following four techniques are currently considered the best practice approaches:
- Generalized Linear Models (GLMs)
- GLM + AI-assisted functionalities
- Pure Machine Learning – mostly Gradient Boosting Methods (GBM)
- GBM + Explainable Artificial Intelligence (XAI) techniques
Similar to Pricing Transparency discussed above, there is no strict definition or a perfect solution for selecting the statistical model. Every single approach has got pros and cons which will be considered below. A detailed analysis of each approach is not expected in this article and will be covered by the upcoming blog posts. Meanwhile, all of those techniques are already supported by the Quantee platform.
1. Generalized Linear Models (GLMs)
This technique has been already covered on our blog [6]. Hence, there is no need to repeat my colleague’s words and describe those in greater detail. However, it is still worth recalling some key takeaways.
First of all, GLMs are well-known within the insurance industry. They have been commonly used for ages with actuaries and pricing analysts scratching their heads about how to polish every single piece of the GLM puzzle. Those years of development mean that there is a common agreement within the industry that they are sufficiently straightforward to understand by both actuaries and customers by e.g. converting them into rating tables which usually satisfies the TCF requirements.
However, fitting a very good GLM model can be an incredibly time- and resource-consuming process and their accuracy might still not reach a satisfying level regardless of the effort put into it. On top of that, there could be a point of no return where GLMs become almost as complex as Machine Learning models. Therefore, it comes as no surprise that a lot of research efforts were put into developing new better approaches.
2. GLMs + AI-assisted functionalities
The availability of Big Data & Machine Learning techniques does not necessarily mean that GLMs are obsolete and will be soon forgotten. It is actually the opposite. Instead of spending a significant amount of time on marginal improvements of GLMs, actuaries are exploring ways of automating the most time-consuming aspects of this approach which would help them gain them more time to fully understand both data they are dealing with and predictions that come out of their models.
These automations are often referred to as AI-assisted functionalities. They consist of e.g.:
- Variable selection
- Optimal binning/spline transformation
- Interactions detection
- Automated category mapping
- And many others
(SPOILER - They will be the main topic of the next blog post so make sure you stay tuned!)
3. Pure Machine Learning
GLM enthusiasts are already aware that there are some new players in the actuarial algorithms league, coming from the world of Machine Learning, and they are slowly gaining more and more popularity. Extensive testing and comparisons seem to point out that in terms of predictive accuracy, those algorithms are usually superior to GLMs [7].

However, they also point out that with a greater degree of accuracy comes greater difficulty of understanding the reasonings behind the predictions. The commonly used black-box reference perfectly captures the main topic of this discussion – with no satisfying pricing transparency levels, using these extremely accurate algorithms could often be found to breach the TCF, PRE and other essential principles of the insurance industry.
Many researchers, such as IFoA General Insurance Machine Learning in Reserving WP members [8], are also exploring other use cases of pure ML applications within insurance business. They prove to be especially useful, where the transparency rules are less strict, e.g. assessing car damage with the use of image recognition [9].
4. GBM + XAI
As the Machine Learning field continues its rapid growth, more techniques of uncovering the layers of black-box become available. Some actuaries who insist on using ML in Pricing would point out that this reference is already becoming out-of-date. At the time of writing this article, a combination of GBM + XAI is deemed to be the best way of taking advantage of a higher level of accuracy provided by ML with no significant loss in pricing transparency. XAI takes inspiration from other branches of statistics, probability and wider mathematics. An interesting approach to explaining ML algorithms is SHAP [10] which originates from Shapley Values commonly used by Game Theory since 1953! Other tools such as Partial Dependency, Ceteris Paribus (single profile analysis), Breakdown of prediction and Model Agnostic Variable Importance are just a few more examples (all of which are available in Quantee platform).
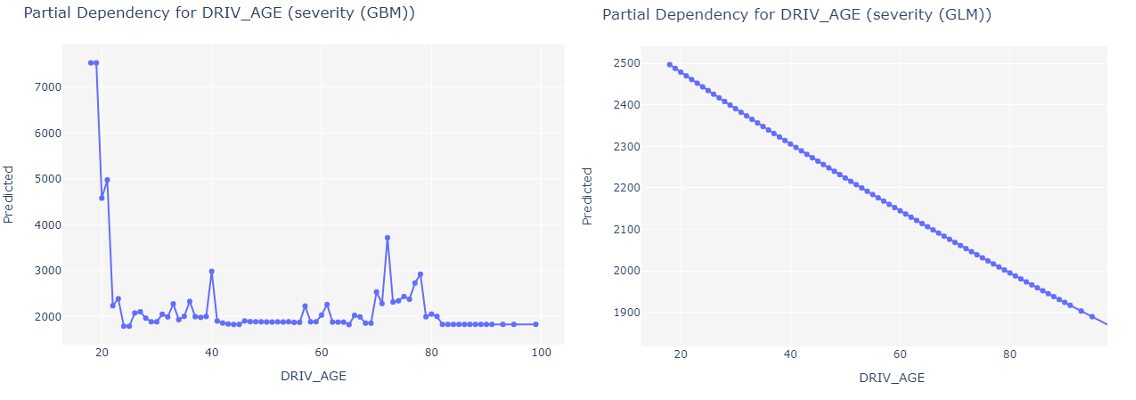
Regulators are constantly considering how to best utilize those in practice. At the moment in time, it still is a very sensitive topic. However, taking into consideration the development pace of this industry, it would not be a major surprise if ML + XAI combinations become widely accepted by the authorities soon.
Summary
The main goal of this article was to emphasize the importance of balance between Transparency and Accuracy in insurance and equip the reader with some tools that are used to ensure this crucial relationship is maintained. In the upcoming blog posts, we will expand on every single technique described above and provide more guidelines on how to utilize them in the best possible way.

In case you find this article useful or inspiring and you cannot wait for the next blog post, please do not hesitate to reach out to us with any questions!
References
[1] EU rules on gender-neutral pricing in insurance industry enter into force
[2] Compilation of Patient Protection and Affordable Care Act
[3] Treating customers fairly - guide to management information
[4] Supervisory statement on differential pricing practices
[5] Discrimination-free Insurance Pricing
[6] How to build the best GLM/GAM risk models?
[8] IFoA General Insurance Machine Learning in Reserving WP
[9] Image recognition in auto damage claim process
[10] SHAP