On maths and ethics - Price Optimisation
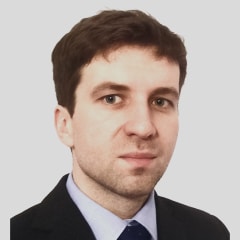

Actuaries by definition are a type of mathematicians who deal with risk measurement and management – an essential skill in the insurance business, which trades risk, or rather financial responsibility for possible negative outcomes of a risk. That responsibility is a product with unknown production cost – and that’s what actuaries estimate, expected loss for an insurance policy is an equivalent to manufacturing costs for any tangible good. However, knowing the production cost alone is not enough to set a sale price – which needs to include some profit margin for a manufacturer or salesmen. Calibrating this profit margin to market realities and consumer behaviours is inherent in every free market commercial trade, both in modern days and throughout history. In insurance (and not only) some advanced mathematical tools have been developed to perfect this process, and it is where actuaries’ maths skills also prove invaluable. Particular nature of insurance business, not only availability of brilliant minds, but also unavoidable need to know each customer and provide them with price individually tailored to their risk, allows for specific techniques of searching for optimal profit margin – which come with their own set of challenges and (also legal and ethical) limitations. We call this process price optimisation, and this article will describe in simple terms its application in insurance pricing.
What exactly is price optimisation?
There’s no universally accepted definition. Even specifically in the insurance pricing context, multiple materially different variants can be found. The American NAIC describes it as the process of maximizing or minimizing a business metric using sophisticated tools and models to quantify business considerations, adding that examples of business metrics include marketing goals, profitability and policyholder retention.1 British IFoA materials define it more broadly as the process of setting prices to maximise a pre-defined measure of customer value subject to a company’s strategic and business objectives2 – not explicitly requiring use of sophisticated tools or models. EIOPA refrains from using “Price Optimisation” term altogether, using “Differential Pricing Practices” instead and describing such practices as: on top of underwriting risk-based actuarial tariffs (expected cost of claims) and other premium adjustments to take into account costs of service (e.g. commissions paid to distribution channels and other overheads like taxes, salaries, cost of capital, etc.), some insurance manufacturers further adjust the premium using a number of different practices which are unrelated to the underwriting risk profile of customers and the cost of service.3
For sake of unambiguity let’s try and define what do we mean by price optimisation specifically in this article. It will be an adjustment of premium, done on top of risk and expense-based pricing process, meant to maximise a measure of company profit, within constraints determined by other business considerations (legal, ethical, marketing or strategic goals).
This may be done manually by scenario analysis, manual changes to profit margin and calculating their impact or by automatic search for optimal price, on which we’ll focus.
Price optimisation requires two inputs:
- Predicted demand (i.e. probability of sale).
This depends on offered premium – it is generally expected to be a decreasing function, the cheaper a product is, the more likely a customer will purchase it. Depending on application this may mean conversion (for new customer) or renewal demand.
- Cost of coverage (risk and any other expenses).
This can be independent of premium or have some premium-dependent components (e.g. taxes) – we’ll for simplicity assume that it hasn’t.
Both are generally provided as models or sets of formulas which allow calculating required values for any given risk profile and depend on properties of that risk (e.g. client’s age, car’s engine power, …)
Optimisation maximises company profit margin, calculated as:
Margin = Premium – Expenses
But that assumes a sale, expected profit needs to take probability of sale into account, hence will be calculated as:
[Written Margin] = (Premium – Expenses) x [Probability of Sale]
It can be reasonably expected that offered price equal to zero guarantees high probability of sale, but negative profit (zero premium and some positive expenses). High enough price would result in a zero probability of sale, hence zero expected profit. Hopefully somewhere in the middle there are price points where price exceeds expenses and probability of sale is nonzero. If so, then there must be a price that maximises expected profit, and in absence of other considerations, price optimisation would be a search for that maximum. In case of multiple client profiles this is repeated for each independently. In case of multiple interdependent products (e.g. Motor Third Party Liability and Motor Own Damage, where probability of sale of one may depend on price of the other), expected margin becomes a multivariate function of each product’s price. Definition of the problem remains the same, it’s a search for this function’s maximum.
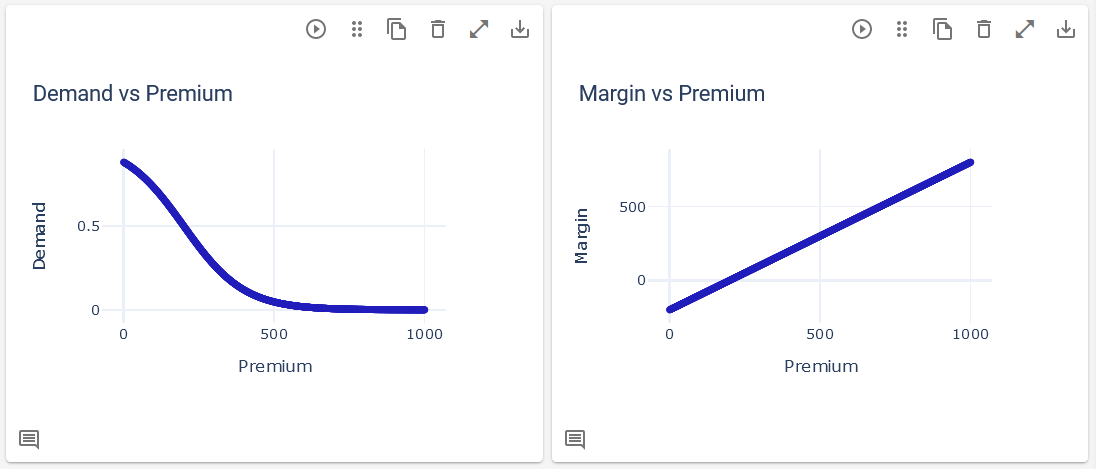
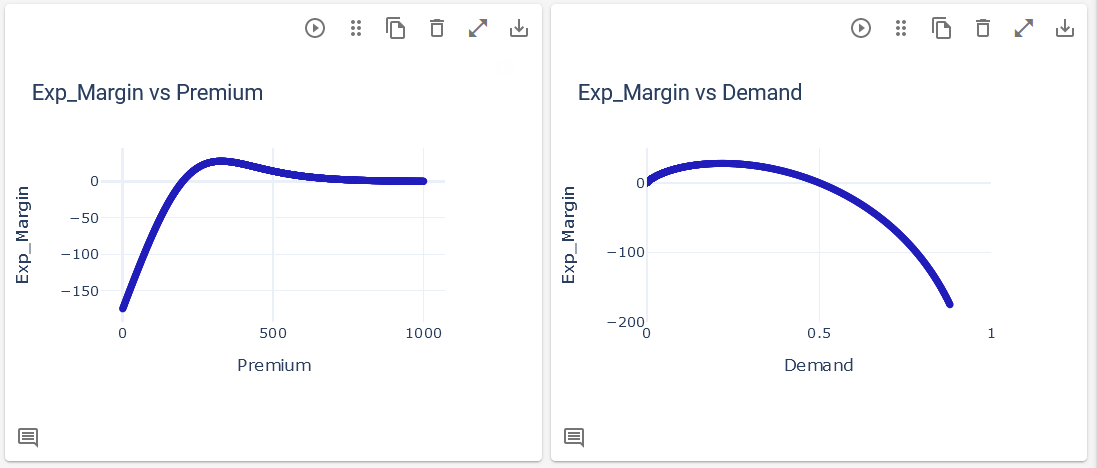
Let’s look back at how we defined price optimisation: “an adjustment of premium, done on top of risk and expense-based pricing process, meant to maximise a measure of company profit, within constraints determined by other business considerations”. We can now see that search for premium that maximises profit has to be done on top of risk and expense-based pricing by necessity. It cannot replace it or be considered an alternative pricing method, just an adjustment. Without knowledge of technical price, expected profit cannot be calculated and its maximum found. With inaccurate technical price or demand model, the optimised price will also not be accurate.
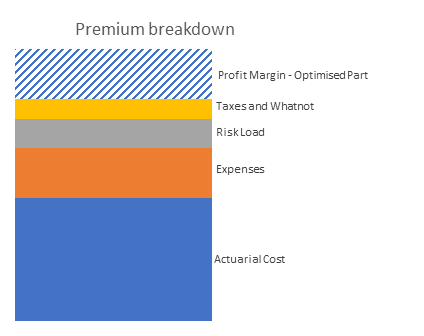
Other business considerations mentioned in the definition are expressed as constraints on the price parameter or any function of price, applied to each client profile independently or to a sum of all. Most commonly, each client’s price is constrained by some minimum and maximum boundaries and whole portfolio by targeted average conversion/retention level. This allows to restrict optimisation adjustments to the level allowable by any legal or ethical consideration, credible demand model predictions range and any other considerations, as well as follow a desired sales strategy, which may focus more on gaining market share than maximising profit.
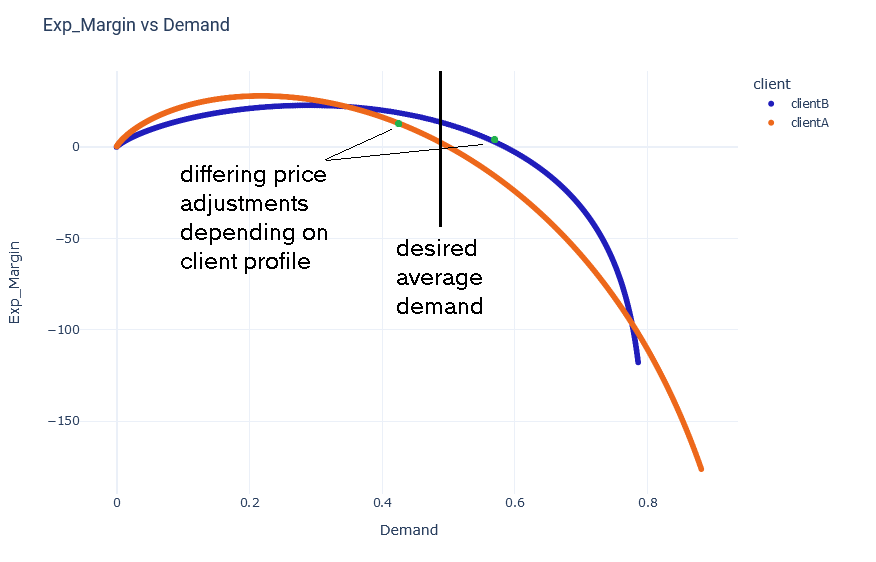
Local constraints (i.e. applied to each client profile individually) do not change much in the process described above. Domain of expected profit function is restricted, and the problem is still a search for its maximum. With aggregative constraints (i.e. applied to globals) the process becomes considerably more complex, as the optimum cannot be found for each client profile independently anymore. Depending on the shapes of expected costs and demand curves, price for an individual customer or a group of customers may be adjusted differently to achieve globally optimal result. This means that a search for the optimal solution becomes a multidimensional problem, with offered price for each client profile being a parameter of a single total profit and average demand functions. Possibly made even more complex with more interdependent products, where even a single client’s offered price is multi-dimensional.
Having performed price optimisation for multiple global constraint values an objective frontier curve can be drown – that is an achievable profit as a function of constraint value. The search for constrained optimum, while complex and computationally expensive, can be done in seconds for portfolio consisting of tens of thousands of client profiles using Quantee platform with its proprietary price optimisation algorithm. In addition, with each constrained calculation, Quantee platform produces an efficient frontier graph, allowing for simple analysis of alternative scenarios and aiding in business decisions. The same is possible (although a bit slower) for multiple interdependent products, also providing an efficient frontier graph for a 2-dimensional problem.
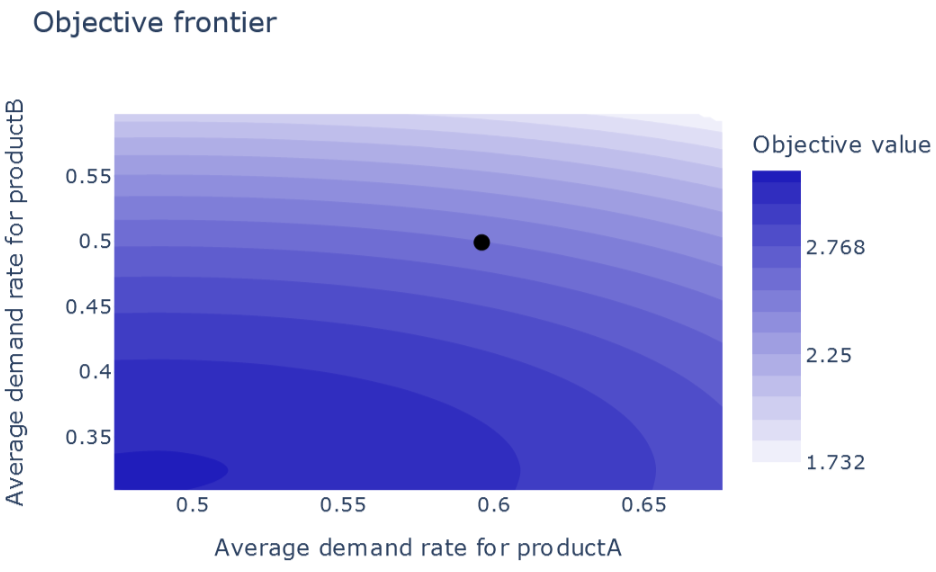
Isn’t this somewhat controversial?
Price optimisation has been a subject of an intense ethical debate. In fact, it is outright forbidden in some jurisdictions around the world (notably some of the US states). Key argument against it is that the same product shouldn’t be sold to two customers at differing price. The same insurance policy may reasonably not be considered the same product when sold to two customers with differing risk profiles. Providing the same coverage to a riskier customer is more costly to insurer and more valuable to policyholder, hence it is fair to offer a higher price to a riskier client and lower to a less risky one. Given the same risk profile, two customers are being sold the same product, possibly at a different optimised price if their demand curves are different. To generalize, customers may be offered a price that is not directly and exclusively linked to their risk profile, but also depends on their individual demand characteristics. Clients with lower propensity to decline a higher price will be offered one. This understandably can be considered exploitation of those less informed, more trusting, less willing or able to challenge what they have been given or shop around and find the best amongst multiple offers.
British Financial Conduct Authority does not ban any and all price optimisation but does forbid ‘price walking’ – which is a particular optimisation practice, increasing price to existing customers vs new business. Existing customers are less likely to compare price offer on renewal to competition and decline an offer too high. It does seem unfair, and potentially bad for business in the long term, exploiting customers trust obviously undermines it.
On the other hand, some other forms of optimisation, for example price dependent on time of purchase (that is increasing price for quotes requested close to start of required cover), seems perfectly fair and likely is acceptable to most customers – it punishes tardiness, not trust. Similarly, if in an effort to gain more foothold in the market a company lowered price not equally to all customers, but to selected groups with more favourable demand profiles – that doesn’t seem particularly unfair. A good indicator of an ethically acceptable practice seems to be whether it is acceptable to a customer who knows about it and understands it, transparency in ratemaking may serve as a good precaution against temptation to increase profits in unethical ways.
Overall, even though price optimisation is specifically banned in some jurisdictions, nowhere are insurers required to base their pricing exclusively on risk assessment, with a fixed profit margin and with no consideration for business strategy or any other factors. As some sources commenting on price optimisation ban in some US states point out – it is a common practice to lower offered prices (relative to risk assessment) for young drivers’ liability cover in order to attract their parent’s business4. This is a price optimisation practice according to our (or British or European) definition – not according to American take though as their definition requires use of sophisticated tools. Our take would be that tools are not good or bad by themselves, more powerful tools simply give us more power and it’s for us to decide what shall we do with it.
According to NAIC task force report on price optimisation5 propensity to shop around is inversely correlated to income, which would mean that optimised price would generally be beneficial to those less well off, which seems fair. There might be exceptions, and it’s for actuaries to identify them and act accordingly. It’s the pricing actuary who decides what factors to consider in demand modelling or how to restrict optimisation for any client groups by their individual price constraints.
Outside of the insurance world, differential retail pricing is used widely (e.g. for Uber rides, holidays, travel and event tickets, or a traditional local marketplace where you can bargain for everything, with initial price often not on display, but given only once the seller sees a buyer and adjusts for their impression) and that is commonly observed as fair, or even beneficial for price-conscious customers as it often allows them to purchase the same product more affordably, at a cost of a bit more time and effort spent shopping around or compromising on some aspect of the transaction, such as buying outside of peak season. It does in most cases differ from insurance price optimisation in that price depends on factors other than specific customer profile, hence extra care needs to be taken in the insurance context to ensure that no customer is treated unfairly.
In addition to all that, monitoring demand and optimised price adjustment may potentially help spotting anomalies in tariff – unusual demand, or a particularly large optimisation adjustment for a particular client profile may mean that competitors asses that client’s risk as considerably larger or smaller which may indicate an error in company’s risk modelling. Even when not actually used in price setting process or used in a restricted form due to legal, ethical or any other concerns, attempting price optimisation can still provide valuable insights. be an interesting and valuable exercise.
Wrapping it up
However complex and not without its challenges, price optimisation can be a very interesting and valuable exercise. With potentially significant benefits to overall income margin and, when done right, no damage to vulnerable customers, company image or actuaries’ souls. For more information or a presentation of Quantee algorithms in use – get in touch!
References
- https://content.naic.org/sites/default/files/inline-files/committees_c_catf_related_price_optimization_white_paper.pdf
- https://www.actuaries.org.uk/system/files/documents/pdf/airey.pdf
- https://www.eiopa.europa.eu/system/files/2023-03/EIOPA-BoS-23-076-Supervisory-Statement-on-differential-pricing-practices_0.pdf
- https://www.iii.org/sites/default/files/docs/pdf/ncoiltestimony-071715.pdf
- https://content.naic.org/sites/default/files/inline-files/committees_c_catf_related_price_optimization_white_paper.pdf
%20(2).png)